Mental health plays a pivotal role in our health and well-being. Nonetheless, mental health is often easily overlooked, as symptoms of poor mental health are difficult to pinpoint. Possibly, this can be even more difficult in case of cancer patients and survivors. In fact, treatment side effects and some types of cancer show symptoms similar to those of depression. Furthermore, diagnosing poor mental health becomes more difficult as face-to-face interaction between doctor and patient lessens.
In this context, FAITH is developing an AI-based supportive tool which will help clinicians in monitoring their patients’ mental health status. Once finalised, the FAITH tool will be a unique support system consisting of two sides: on one hand, a mobile App (supported by wearables) interacting with patients and gathering data on their status; on the other, a Dashboard for clinicians which will allow them to consult patients’ data, providing predictions on users’ mental health conditions. Currently, during the ongoing trial phase, FAITH is training its algorithm to recognise trends and fluctuations on patients’ data. Simultaneously, the Clinician Dashboard also functions as a monitoring platform to check data quality. This is key to ensure that the algorithm learns from good quality data, and consequently will be able to detect downward mental health patterns, once the solution will be ready for clinical use.
Hence, at this point of our project, one can see FAITH as a coin with two faces: one engaging patients and gathering data, the other interacting with clinicians, who can check the quantity and the quality of the data from patients. In this light, the Trials’ Clinician Dashboard fulfils an important task. As the these data will train FAITH solution’s algorithm, it is crucial that their quality is good. In fact, an AI and its algorithm are as only good as the data they were trained on.
This article will explore the FAITH solution from the clinicians’ perspective, specifically the trial phase version of the solution. Furthermore, the article will expand on how User Experience Design (hereafter UX Design) improves Human-Machine Interaction (HMI), supporting data quality.
FAITH Data Collection
A reliable AI model, able to detect symptoms of depression and anxiety,\ depends of course on good data collection. To carry that out, there are several steps. Most importantly, a clear identification of the right data to acquire to keep track of relevant variables is central. In FAITH’s case, these are depression markers. The FAITH protocol states the data to be monitored, how they are monitored, and their use in mental health monitoring.
To ensure effective data collection, FAITH combines passive digital tracking (i.e., sleep and physical activity monitoring) and proactive user engagement through medical questionnaires (i.e., food and water intake and Quality of Life). Unsurprisingly, also the FAITH mobile App has its own UX Design to ensure patient engagement and data quality.
But what about doctors? Even for them, usability and user experience are key to building a relationship of trust.
Building an Inclusive User Experience flow: the clinician’s perspective
To build a functional UX Design, the starting point is to define users and their needs. In FAITH’s case, these are cancer patients’ healthcare teams. Indeed, they need easy access to the data collected during the trials from the FAITH mobile App. Moreover, as FAITH gathers a vast amount of data, and clinicians need to fulfil a two-fold role consisting in checking data quality and retaining awareness about which data is going to actually shape the AI model, the current trial version of FAITH’s solution responds to their needs, facilitating their crucial task. Hence, the FAITH Trial’s Clinician Dashboard.
The FAITH Clinician Dashboard arranges the collected data in such a way as to facilitate access. Furthermore, it also facilitates user/App interaction monitoring, as regular engagement is necessary for good data quality.
FAITH Clinician Dashboard
The figure below shows the FAITH Clinician Dashboard Homepage.
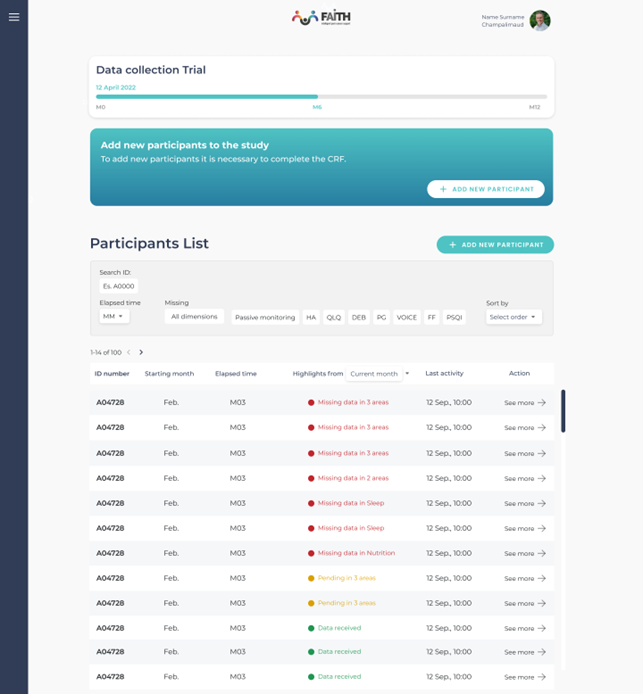
Figure 1: FAITH Clinician Dashboard Homepage
Patients are anonymised with ID numbers. From a first glance, doctors can have an overview of their interaction with the FAITH App. This is evident in the columns reporting for how long they’ve been using the App. The columns also highlight their data status and the time of their last activity. Overall, the Dashboard presents the data in a way that helps clinicians identify users who are providing data inconsistently, and thus need more engagement. Here, UX Design not only aids doctors in navigating data. In fact, it is critical to provide a way to check data quality by monitoring patients’ engagement.
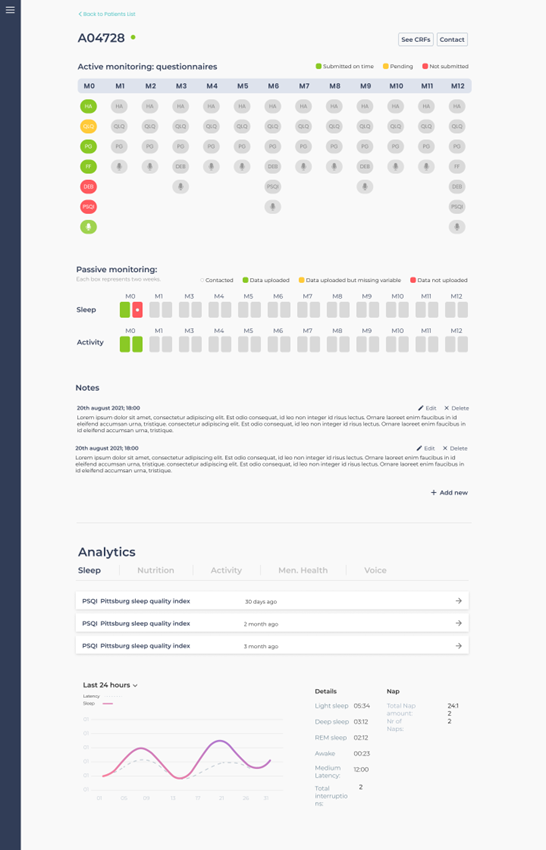
Figure 2: Clinician Dashboard single participant overview
Figure 2 shows the overview page of a single user. Users’ personal pages feature each patient’s data, their engagement with the App, potential gaps in data acquisition, and depression markers analytics. The analytics feed in a clear way crucial information about patients’ performance in five depression markers identified by FAITH. Specifically, these markers are Sleep, Nutrition, Activity, Mental Health, and Voice. By closely monitoring the depression markers, doctors will be able to have an insight of patients’ data quality. In addition, they also will be able to check the regularity of App/user interactions.
In its final version, the FAITH Clinician Interface UX Design’s objective will be to facilitate doctors’ and healthcare professionals’ assessment of their patients’ health. Indeed, FAITH will provide doctors with powerful means to monitor patients without face-to-face consultations. The AI will train on the trials’ data to recognise fluctuations in the patterns of patients’ psychological outlook. During this data collection phase, it is vital to keep track and monitor what information reaches the system, and consequently, on what data the AI will train on. This is pivotal for enabling explainability and transparency of the results when the AI will be introduced.
In fact, during the FAITH trials, besides interacting with the FAITH App, participants will engage in regular face-to-face psychological consultations for mental health clinical assessment. This will provide a comparison of the patient’s outlook with the collected data. Furthermore, it will help identify models for the algorithm on which to analyse data, and eventually fine-tune the algorithm itself on certain patterns.
User Requirements and Information Architecture
The team building the Clinician Interface is working closely with healthcare professionals to understand what they expect from FAITH. Indeed, the Dashboard and its Information Architecture (i.e., the way of organising, structuring, and labelling content in an effective and sustainable way) are tailored to the doctors, their priorities and work practices. For instance, realising that not all trial participants would start at the same time, developers needed to adapt the Dashboard in a way that doctors could clearly check at which point of the trial each participant is, their engagement level, and even if missing data is due to poor engagement or App/wearables technical issues. The single participant overview allows clinicians to be able to quickly spot which patients are not providing data to contact them and understand their reasons.
Another example of the application of UX Design to the Clinician Interface is data visualisation. FAITH collects a great number of different types of data: from quantitative, passively collected data, to qualitative, actively collected data from seven different questionnaires in the FAITH App. Furthermore, each type of data comes in with a different frequency (some weekly, others monthly). This makes it difficult for analysts to understand data and engagement quality, and most importantly, which data is missing. As a solution, the Dashboard is working on a visualisation mode that displays all data in one page (fig.2). Also, colour coding is designed to present data collection status, while quantitative and qualitative data are clearly divided to ease the page consultation.
This is the result of many iterations and exchanges between the hospitals, the developers and the UX designers.
UX Design: why does it matter?
A well-constructed UX Design can provide a solid support to clinicians. Most importantly, it facilitates the navigation of data, enhancing and simplifying their analysis. In the Clinician Interface final form, the Dashboard will actively support healthcare professionals in monitoring patients’ mental health status. Such feat will allow them to remotely check on patients and adapt their treatment when needed. Indeed, UX Design can effectively save doctors hours of work on skimming through data by organising, presenting, and filtering it at will.
From this perspective, UX Design plays an essential role within the FAITH environment. It doesn’t only support doctors, but is also the enabler for transparency and explainability, allowing to monitor data quality. This is key for training the AI behind the FAITH solution.
Ensuring FAITH’s reliability
The FAITH Clinician Interface is a brilliant example of how digital healthcare can employ UX Design to support clinicians. The trial version of the Dashboard and its related Information Architecture main objective is to visualise data in a way that doctors can have a clear overview of their patients’ status and assess the quality of the data they’re providing. The FAITH tool means to help clinicians by creating a unique channel of communication between patient and healthcare providers. FAITH will allow to keep a close relationship between the two, even if remotely. Building the solution around the people who will use it and keeping their needs at the centre are the best guarantees not only for FAITH’s success, but also for eHealth and healthcare’s digitalisation.
Once the trials are completed and the FAITH AI algorithm is adequately trained, the next steps will focus on designing the Experience and User Interface for visualising the AI results predicting the mental health fluctuations. This will support transparency and explainability, leveraging exactly on the awareness built during the data collection stage.
In its final iteration, the FAITH tool will be able to monitor patients’ mental health status. Furthermore, the AI will be able to predict fluctuations, providing early warnings in case of negative trends leading to mental health deterioration.
Authors: Vera Ferraiuolo, Giuseppe Frau, Alessandro Tedeschi Gallo, Tommaso Vendruscolo – Deep Blue